Teaching AI to Save Humanity with Buster Franken of Fruitpunch.AI
Arjé Cahn: What if we could harness
the most powerful AI technology.
Not to maximize profit.
Profits or boost engagement, but to
solve humanity's greatest challenges.
Today's guest Busta Franken.
Is doing exactly that.
As the founder of fruit punch that AI
Buster has built a community of 4,500
AI engineers who are using your skills
to tackle everything from environmental
crimes to endangered species protection.
Their projects have exposed industrial
polluters, revolutionized wildlife
conservation, and are now pushing
the boundaries of medical research.
But this isn't just
another tech success story.
It is about a fundamental shift in how
we think or should think about artificial
intelligence and its role in society.
While tech Gines focus on growth metrics.
Buster is training the next generation
of AI engineers to put impact at
the heart of everything they do.
Record it by the warmth of a
woodstove in our forest cabin.
This intimate conversation reveals how
a growing movement of technologists
is choosing purpose over profit.
You'll discover what drives D's engineers
to tackle humanity's biggest problems
and hear about Buster's ambitious plan to
mobilize a million more to join the cause.
So join us for a thoughtful discussion
about using the world's most powerful
technology as a force for good.
Hey, Buster.
Hey, good to see you again.
Good to see you.
Thanks for joining us today.
Absolutely.
My pleasure.
And we're sitting in the forest,
we're sitting inside, no, no campfire.
Buster Franken: Yeah.
We're afraid of the wolves.
So, yeah.
In the dark.
We want to leave them in peace.
So we go back in our little hobbit hole.
Arjé Cahn: Well, I hope it's nice
and comfortable sitting next to the.
Buster Franken: It is very comfortable.
The
Arjé Cahn: wood stove.
Buster Franken: Yeah.
Yeah.
Arjé Cahn: Good.
We're talking about Fruit Punch
AI and, and your personal journey.
There
Buster Franken: we are.
Arjé Cahn: And, but, but before we
start talking about your thing, um,
can you help us understand the scale
of what Fruit Punch AI does today?
Buster Franken: Absolutely.
Um, so pretty much is a global
community of AI engineers, about four
and a half thousand AI engineers have
worked on various projects that we
have organized, um, might look like
Kaggle for people that know that in
the beginning, uh, but, uh, really our
challenges are collaborative projects.
So people work in a team
of around 30 AI engineers.
They work together to solve
a problem within 10 weeks
for an impact organization.
And these four and a half thousand
engineers have worked on over 40
different challenges and teams, uh,
with organizations like European Space
Agency on detecting and, um, and, uh,
mitigating forest fires to Stanford and
Colgate University to identify elephants
in, uh, in the Congo to medical centers
to help save lives by detecting diseases.
Um, yeah.
That's a little bit of an
idea of the, of the scale.
And of course the, the scale of
the impact is the follow on effect
as well as solving these problems.
So to give you an idea of a specific
challenge, uh, we worked with green
peas to detect illegal toxic clouds
coming out of a steel factory.
This was a problem in the Netherlands.
We do challenge all over the world,
but this, uh, was in the Netherlands.
It was a local one.
It was a local one.
And they had been in this dispute.
Uh, about, uh, you know, if they were
actually emitting, emitting toxic
clouds and it was hard to prove.
So at a certain point they started kind
of crowdsourcing, um, uh, the detection
of these toxic clouds, but it was very
hard to verify just with human power.
So we built AI models that
surveyed or that, um, surveillanced
the factories at all times.
And within, uh, I think it was three or
four months from that, they had accrued 4.
1 million euros in fines due
to the models that we created
that observed these factories.
And eventually it led to a decision
by the Dutch government, by the
sitting party at that point.
Thanks.
Thanks.
To actually officially externally monitor
these factories, um, which makes that they
can't emit these toxic clouds anymore.
And that, that's thousands of tens of
thousands, if not hundreds of thousands
of people that, uh, that had incredibly,
incredibly Heightened risks of cancer.
Hmm.
Um, and the goal, of course, is to then
be able to, we open source everything
to then be able to roll this out to
other places in the world as well.
Arjé Cahn: So, hold on.
So you, you said you
crowdsourced, uh, the, the clouts.
The,
Buster Franken: yeah, the,
yeah, the detection of, of
the, of the, of the clouds.
Yeah.
What do you mean with that?
So, so these were, you had these stokes,
uh, uh, you know, it's a kind of, um, kind
of CEO buildup in these, uh, in these.
Factories that can come out in
these fumes if the plant is not
regulated thermally, optimally.
Okay.
Um, so they have a responsibility to
do this optimally, but for all kinds
of reasons, they weren't doing this.
And then, uh, when this actually
comes free and goes up into the
atmosphere, you can, you can kind
of see it and experts can see it.
Right.
But then, you know, having experts
monitoring multiple of these
exhausts for 24 7 is very difficult.
Arjé Cahn: Because they have
multiple of these fumes.
Yeah,
Buster Franken: yeah, yeah.
Multiple of these exhausts.
Yeah, chimneys, basically.
Yeah, exactly.
So, so that's where the
power of AI comes in.
Arjé Cahn: Okay.
So this is how you got to the, the,
the green peas challenge, um, to detect
these clouds and, um, or, or not detect
the clouds to monitor the clouds.
Uh, but you did many others and
one, one that really, um, got
me excited maybe because it's so
cute is the, uh, is the seals one.
Buster Franken: Yeah.
Facial recognition of seals.
Yeah.
Arjé Cahn: Right.
And this, this blew me away
because you, uh, apparently.
You can use AI facial recognition to
separate one individual seal from another.
Yeah.
Right.
These are seals, right?
Yeah.
Yeah.
And it just works.
Yeah.
Right.
Yeah.
Absolutely.
Yeah.
Kind of, it kind of makes sense if
you think about it, but for us as
human beings, they all look the same.
Buster Franken: Yeah.
But we're very geared with our recognition
model works on other human faces.
Yeah.
So, so the, so, but essentially there's.
Why would
Arjé Cahn: you want to recognize one seal?
Buster Franken: Yeah.
Why is that important?
That's this one is about, um, uh,
it's about ecosystem management
or, or, uh, observation.
So these seals mostly living, um,
with the universities that we were
working together with, um, I think
this was, I think this was Colgate.
Arjé Cahn: Okay.
Buster Franken: Where
is Colgate based out of?
New York, I think.
Yeah.
Actually, that's a good question.
Yeah.
We're always, we're always working online.
So you don't even know.
So I'm not, yeah, I'm not 100 percent
crystal on where exactly they are.
But they're pretty smart.
They're pretty smart.
Yeah, yeah, yeah.
And they, they, so these are marine
biologists that are monitoring seal
populations because seals are at the
top of the food chain or somewhere
at the top of the food chain.
So they, you know, they eat the
fish and then if there is something
wrong with You know, even the,
the plants locally, then this will
translate into there being a lower
fish population, which will translate
into the seals not doing as well.
Right.
So, so basically you can get a very,
they're a great indicator for how the
ecosystem, the whole ecosystem is doing.
So that's why it's really
important to monitor seals.
And that's why you generally see that
the, the, that there's a lot of emphasis
on monitoring predators and ecosystems.
And, uh, for this, you need to
know not only how many seals
there are, but also, you know, how
many new seals are they making?
Um, how fast are they dying?
Is that at a normal rate?
So you need to be able to
detect individual seals.
Arjé Cahn: Yeah.
Buster Franken: So they actually,
these marine biologists, they, what
they used to do is they take pictures
on the I think this is the East coast
of the U S to take pictures of these
seals, uh, sitting on the rocks.
And then these marine biologists,
they go through every picture
and they have every seal marked.
And they're like, I think
this is Henry to seal.
And then they pick, take a picture from
the database, which is Henry to seal.
And then they're like,
yeah, this is Henry to seal.
And then they annotate.
Like all these different seals that
they see in the picture, and then
they do that for every picture.
So time consuming.
Yeah.
So this takes, this takes like, you
know, three hours per picture, right?
Right.
And after we were done with
the challenge, this took 2.
7 seconds for a picture.
And so I'm
Arjé Cahn: actually quite surprised
that these people are able to
recognize these different seals.
Buster Franken: Yeah, yeah, no, it's, it's
impressive, but they're trained biologists
that they know what they're doing.
Yeah, they know what they're doing.
They know their seals.
Yeah.
Yeah.
And, uh, but, but this is, yeah, this
is, this is what we did with them.
And, uh, yeah, it's very important.
So
Arjé Cahn: where did
these pictures come from?
Uh, so
Buster Franken: they take
them as marine biologists.
They, they are assigned to
monitor a certain ecosystem,
uh, along the coast there.
Uh, so a certain, certain spots that they
know as a breeding ground, for example.
And then they go at a fixed time
of year and they take pictures.
Right.
You go out on their boat to the rock.
Arjé Cahn: Okay.
And then, um, okay.
So you, so you got the face
recognitions for, for seals.
You did that.
Buster Franken: Yeah.
Arjé Cahn: Was that
the first facial, like.
Wildlife facial recognition
challenge that you did.
Was it the first, that's a good question.
Where like after that one,
you had bears, turtles,
Buster Franken: sea turtles,
Arjé Cahn: and a whole
bunch of other ones, right?
Yeah.
So you got your community got
really good at recognizing.
Yeah.
Buster Franken: Uh, individuals.
Yeah.
Well, life identification.
That's a, that's a big one for us.
And that is in the, in the coming,
in the coming year, we want to, we've
identified a couple of focus areas like
this, where we really want to hone in on.
And this is really one of the things
that we just got incredibly good
at in the, in the world, at the
world stage of doing these things.
So seal nets.
Uh, for which what's
Arjé Cahn: the world stage of face right?
Animal face.
Yeah.
Buster Franken: It sounds a little
bit like a, like a fetish or something
like a very niche, uh, Reddit group.
Arjé Cahn: Did they come together?
Meetings?
Buster Franken: No, no.
But it's rather, it's
rather just all these.
All these marine biologists and biologists
that are, um, you know, they're realizing
that they need to, that they can use
AI for this, but they don't get any
training in, in AI or in machine learning.
So they're, they're, some of them kind
of try to teach themselves and it's
like, they try to hack stuff together,
but it's, it's really a place where
you can make a lot of impact with,
with, uh, the model that we have.
Um, especially because yeah, this is
a place where there's, there's not
necessarily a lot of money to be made.
But, um, but there's a huge positive
impact to be able to monitor
these, these, uh, these ecosystems.
Can you.
Arjé Cahn: So let's talk a little bit
about the impact that it's creating.
Buster Franken: Yeah.
So, so in, um, to be able to,
this is, this is a major one.
I think this is the biggest one.
So of course you can talk about the
conservation of this specific animal,
but, um, but with these predators, yeah,
yeah, well, no, with the group, you know,
like the seals or, or these sea turtles.
All right.
What the fact that there
are Keystone species.
So, so this, this predator that is
indicative of the entire ecosystem means
that you can, you can, if you, if you
see how they're doing, then you can say
something about how the ecosystem is
doing, which you can take to, um, people
that are locally like factories, for
example, that are, uh, polluting and they
might go over, over a certain limit or the
limit might be, you know, too, too high.
And then you can, you can take this
information, this actual data about
like, no, but over time, there has been
this change to actually go to local
government and as evidence and say,
like, this needs to change in this way.
So did that happen?
Arjé Cahn: Yeah.
Buster Franken: With
Arjé Cahn: one of these challenges.
Buster Franken: I, I haven't got
like a direct report back that, that,
that, that, you know, that they did
like had like a lawsuit or something,
but this is generally their goal
with this, so, so I'm, I'm guessing
they're doing this all the time.
Arjé Cahn: Yeah.
Yeah.
Do you have an example of
another challenge where you
created very tangible impact?
You mentioned the one with the clouds,
of course, which was a real success.
Buster Franken: Yeah.
Arjé Cahn: Um, are there others?
Buster Franken: Um, yeah.
I mean,
I'm kind of, I'm kind of blanking now
that, that you're asking me like one.
Arjé Cahn: Okay.
Maybe we'll, maybe we'll get,
maybe we'll pop up later.
So, so let's zoom out a
little bit first before we go.
Um, so Fruit Punch.
Buster Franken: Yeah.
Arjé Cahn: Is a community.
Buster Franken: Yeah.
Arjé Cahn: 4, 500 engineers, you said,
and you're solving these challenges.
Somehow you're, You're
collecting challenges.
Yeah.
You have a way to find them through
the community or other ways.
And then you work with a
university or with, um,
Buster Franken: government, governmental
agencies, or impact startups.
We work a lot with impact startups.
Arjé Cahn: Right.
So, um, so it's, it's
pretty, pretty powerful.
What, why are you using the frame
challenge for these projects?
Buster Franken: Yeah, I think it, I
think it comes from that, you know,
everything we do is always geared towards
the sustainable development goals.
So we just took, we just took a couple
of, we made a couple of decisions in what
we are and what we aren't going to do.
Right.
So we're not going to define what is good
and what is not good in a broader sense.
So I love these philosophical topics,
but we just said like, okay, the,
the UN sustainable development goals.
That's what we're optimizing for.
Yeah.
So, so we take on any challenge
that hits on, that hits on one of
the sustainable development goals.
Although there are sustainable
development goals like, um, like, uh,
industry and innovation, which It's not
necessarily something that we focus on.
So we focus more on life on land,
life below water, um, zero hunger, uh,
equality, um, and then, uh, that's, yeah,
good health and well being, like, so
mostly health care, um, climate action.
So health care, climate,
biodiversity, and social issues.
Those are, those are our main, uh, areas.
Arjé Cahn: So far.
There he is.
Those are the challenge, the
kinds of SDGs that challenges
have been, um, pointed towards.
So can, can anybody just come to you,
to your community and say, Hey, you
know, um, I want to dig into this.
I have a dataset and, uh, I want to
understand X, Y, Z, uh, from this dataset.
Can I just bring it to you?
Buster Franken: Yeah, you can definitely.
And, uh, please do if you're, if
you're, if you're watching this, if
you're listening to this, um, so we
are quite critical on what kind of
projects we take on because we want
to make sure that we make a maximum
positive impact with every project.
So that means that next to
that, there needs to be.
A data set, or sometimes, sometimes
there's public data available, right?
But then, but then it would be nice if you
have some like concrete idea about this.
Does
Arjé Cahn: it always start with the data?
Is that the way to go?
Buster Franken: Um, it usually starts
with the data because, uh, with the
data and a certain problem, right?
So, um, but the, but the thing is
when, when you, when you start with,
uh, just any problem, there's a lot
of things that aren't necessarily,
uh, suited to be solved with AI.
Okay.
Right.
So some things, some things just need
to change in terms of government policy.
And that's not what we do.
You know, I have definitely thought
about like, Oh, somewhere in the
future, it will be nice to expand
to other domains and maybe do like,
Oh, you have a certain problem.
And then it turns out that the
most impactful thing that you can
do is just, uh, Uh, like, uh, put
on like a huge lawsuit against
a certain, a certain company.
That would be cool, but it's absolutely
not what we're doing right now.
But other people are doing that.
Other people are doing that as well.
Yeah.
Yeah.
So, so we focus on things
that can be solved with AI.
So it's, it usually starts with
the data and then a certain
problem that needs solving.
And what we Kind of the, the first thing
that we look for is that it's a problem
that's really core to the organization
that's bringing us the, the, the, the
problem or the potential challenge.
So there's a lot of individuals that
would love to, they're like, Oh,
but, but this is a huge problem.
And then we're like, yeah, absolutely.
But we are not domain experts in, you
know, in, in some, some specific, you
know, uh, type of disease, for example.
Right.
And we don't have access to
patient data and these things.
So that's generally why it
starts with the data, because.
Because that means if an organization
has access to the data and is motivated
to solve the problem, then they're,
they're in a position where we can also
actually help them and make a big impact.
Arjé Cahn: And then,
and then what do you do?
Do you, you, you like who comes up
with the definition of the challenge?
Buster Franken: Is that you?
Yeah, it's usually us in collaboration
with what we call the challenge owners.
Uh, us as my, my little
team of, uh, bandits.
Yeah.
Yeah.
So, uh, so that's me and, uh, and,
uh, Dorian, our challenge manager.
And then we also, uh, on a project
basis, hire the best experts
from our community to come in.
So for example, We're doing, we're setting
up an, uh, AI for genetics research
challenge with Phillips right now.
And, um, they're
Arjé Cahn: the industrial company.
Buster Franken: Yeah.
Yeah.
They're doing a lot of medical
care, the medical health
Arjé Cahn: care appliances.
Buster Franken: Yeah, exactly.
And.
There we hired somebody from our community
that is a, an associative, uh, or a,
uh, associate professor, uh, that has
a lot of experience in bioinformatics.
Arjé Cahn: So this is
someone from the community.
Yeah.
Buster Franken: Yeah.
Arjé Cahn: Cause you already know.
Yeah.
And now, but now you're paying them.
Buster Franken: Yeah, so we're paying
them, uh, you know, it's, it's not, uh,
a similar amount to, to, you know, what
you would normally pay an AI engineer.
Okay.
Um, but it's really, it's really,
yeah, kind of to, to, to make it fair.
So we, so we try to compensate them,
but, uh, but yeah, the, the challenges
are the, Not for profit part.
So, um, so we, uh, we try to
keep the costs low for the impact
organizations that we work with as well.
Arjé Cahn: We should talk about the, the
other side of it, the for profit part.
Buster Franken: Yeah.
Arjé Cahn: Um, how, how does that work?
Because you've got, you harvest
these challenges and you have the
community execute on the challenges
in, in the not for profit.
Buster Franken: Yeah,
Arjé Cahn: part of the business.
Buster Franken: Yeah.
Arjé Cahn: Apparently there's also
a profit part of the business.
What does that do then?
Buster Franken: Yeah.
Yeah.
So what we, we, of course, when we
first attracted investments, you
know, we, I started fruit punch as
an NGO then, uh, was a certain point
approached by investors who saw kind
of the growth of the community and
the things that we were doing and,
uh, decided to turn it into a startup.
So then.
With the specific idea of like, I want to
make this sustainable and scalable, right?
And for that, you need to find a
business model that actually works
so that you can be self sustaining.
Um, so I really believe in this way of
doing business instead of, um, asking
for a donation and then being able
to do one project, you know, you can,
you can ask for an investment and then
look for a business model with which
you can 10 X, a hundred X, a thousand
X, your impact at a certain point.
So,
Arjé Cahn: because
that's the thing, right?
You want to generate
that additional impact.
Buster Franken: Yeah, yeah, exactly.
So, so it's, to me, it's all about
getting to this position of leverage.
Right.
So, um, and that's also why I found
the startup path really interesting.
And then what we noticed at a
certain point is that actually the
education It has been, um, something
that we promoted since the start.
We actually, the first thing that we
tried was going to the universities and
saying like, Hey, uh, don't you want to
have your students participate in our
challenges as challenge based learning?
Because we really believe that the future
of education is, you know, on the one
side using, uh, things like chat GPT
and AI in the best way possible to,
to help people learn, um, but also.
You know, not go fully digital because you
need to be put into real world scenarios
where you're actually, you know, where
you're dealing with a real stakeholder
with dirty data, where you need to solve
an actual problem to actually learn and
cement things into your, into your memory.
Turn them into experience.
Arjé Cahn: Is
Buster Franken: yeah,
Arjé Cahn: the right
approach to education.
Buster Franken: Yeah.
Yeah.
No, very strong.
And there's a lot of research
supporting this as well.
So at the university where, where
I studied technical university of
Eindhoven, uh, there's a lot of
research being done into challenge
based learning specifically.
So that's also where I kind of,
where I borrowed the term from.
And, um, back then Isabel, the professor
that was working on this, uh, we had
regular conversations at a certain point.
She told me like, yeah.
We tried, we put a PhD student on trying
to make a business model out of challenge
based, uh, education didn't work.
And I, and then I said like,
well, watch me and we'll talk
in a few years and then yeah.
So, so I kind of, that's, so it's
always been a very important part
of what we're doing, but then in the
beginning universities, We couldn't
take it past the stage of like, uh,
you know, a pilot project because
of course it fundamentally changes
the way that we're educating people.
Right?
So it's not, it's not
evaluating people based on a
Arjé Cahn: test.
So, sorry, I just want to make
sure, um, we all understand
what you're talking about.
So challenge based learning means
that you don't just get a normal.
Assignment, right.
One off the shelf that the professor
has been using in the past couple
of years, but you, you get, you
get thrown into the deep end.
Buster Franken: Yeah.
Real problem with
Arjé Cahn: a real problem with dirty data.
Buster Franken: Yeah.
Arjé Cahn: Real stakeholder.
What do you mean with dirty data?
Buster Franken: Dirty, dirty data is
like data that hasn't been cleaned.
So my data data cleaning is.
You can have both.
You can have scores, you
can have different points.
You just have the good values.
You can have
Arjé Cahn: scores but
they're not always the same.
You know, yeah.
unless you, you need to be
able to, to get a good score.
it's it's all about the operations,
that's tracking everything, Yeah.
if you get a
Buster Franken: good score, All
Arjé Cahn: right.
The challenge is figure
all these things out.
Buster Franken: Yeah, exactly.
So, and they need to, they need to go
into conversation with the stakeholder
as well and ask them questions.
So the, the information retrieval
there is not, uh, you know, is
not in a, in a written assignment.
But is in, in the way that it would
go in a real world situation as well.
Somebody has a problem and
you need to figure out how to
solve that problem for them.
Like I
Arjé Cahn: mentioned, it's very
hard for people to come up with the
right data, but to collect the right
data, like when you start, yeah.
And, and it's all messy and it's,
it's, so, so this is the, the for
profit side of the business is your,
your, um, you're selling education in
a way to universities or, or schools.
Buster Franken: Enterprises.
Enterprises.
Arjé Cahn: Okay.
Also, also the companies.
Buster Franken: Yeah.
Yeah.
So actually, so actually mostly, so
this is like selling to universities.
The first thing that we tried and
then we moved on and then we tried
a lot of other different business
models like recruitment and, and like
a kind of gig economy, uh, platform.
But, uh, in the end we got back
to education because while we were
doing these challenges, we kept being
approached by companies that were
like, Oh, I want, can our engineers
participate in your challenge, but then
work on specifically the MLOps part.
And can they be in a team together?
So we have a little bit of team building.
So we just started asking money for this.
And then at a certain point we were
like, Oh, wait, people actually want to.
Pay to be, to, to participate
in our challenges.
So it's like the exact opposite.
So people usually ask me first, like.
So do you pay these people that
participate in your challenges?
And now the reality is that we have
some volunteers in the challenges,
but we have people paying to
participate in the challenges,
Arjé Cahn: but there's a
different skill level as well.
Yeah, exactly.
The volunteers are.
Experts and then the others,
the youngsters are paying.
Buster Franken: Yeah.
And the youngsters are, are people are
people generally, you know, there they're
people that already already professionals.
I would say already programming
professionally, but then are getting
into machine learning or, or want
to upskill in a specific skill.
So we have a lot of media people as well.
Um, yeah.
And they then pay to, yeah.
Also get a little bit of extra guidance,
uh, from, from the experts on our team
or people that we, experts that we pay
from the community to give this guidance.
Arjé Cahn: So if you're an AI engineer or
an engineer who wants to get into the AI
space, um, you want to provide them with
a place that they can go to, to apply
their skills, do some good for the world.
And then change the course of humanity.
Is that what I just heard you say?
Buster Franken: Yeah, exactly.
Yeah.
Yeah.
And we also provide, you know, we
also provide courses and, and live,
live programs for people to actually
learn how to apply AI, right.
And, uh, a platform where they can
interact with a lot of other engineers
and, and, uh, and improve their skills.
Why do we need
Arjé Cahn: to change the course of AI?
I'm sorry, of humanity.
Like, I'm just thinking about that
word, like the, the weight of it.
You need a million engineers.
to change the course of humanity.
Why do you say that?
Buster Franken: Yeah.
Why do I say that?
Or why do I name, why,
why do I put it like this?
Why this number?
Humanity.
Humanity.
Why humanity?
Yeah.
Um, yeah, because I think
we're the dominant species.
You
Arjé Cahn: didn't say change the world.
You said change the direction.
Buster Franken: Oh, that's okay.
Now I get it.
Yeah.
Yeah.
No, that's a good point.
Um, yeah, because Because
I, because I think, yeah,
I mean, to me, it might seem very
obvious that, that like the path that
we're on right now is just not, it's
not going in the right direction in
terms of climate change and biodiversity
loss and, and, and all these things.
Um, and I think, I think it's,
I think it's about, it's kind
of about humanity growing up.
Right.
So, so I see these in
the same way that one
Arjé Cahn: responsibility,
Buster Franken: yeah.
In the same way that, that an
individual goes through various
stages of development, right?
First you're a child, completely
egocentric, very cute, but
I think egocentric, right?
That's like, yeah, of course.
And, and then the teenager and then,
and then, you know, you, then you, you
want to do whatever you want and you're
not listening to everyone, anyone.
And then, and then at a certain point,
you know, you're an adult and you need
to get some like emotional intelligence
and be responsible for your actions.
And I think this is also what's happening
with humanity kind of like this.
I call it the, uh, or it's a, it's a,
it's a, it's, it's a known trope, the,
the, the fall from paradise, right?
So we've been falling from paradise
ever since the, the agricultural,
uh, and then industrial revolution.
Um, where, where there was a point at
which we were just one of the animals.
We were completely, you know,
little child, basically we were
completely, we could do whatever we
wanted and, and, uh, and yeah, we
were just part of this ecosystem to
Arjé Cahn: allow ourselves to be selfish.
Buster Franken: Yeah, yeah, exactly.
Exactly.
And then, and then at a certain
point we started to have.
We started to, uh, yeah, use, use
tools and use our intelligence more
and more to shape the world around us.
And with this came knowledge and,
and, and this is also the, the
biblical fall from paradise, right?
So some knowledge is introduced with this
shame, uh, with this hierarchies, with
this war and disease, because cities were
built and, you know, all of these things.
And then Um, and then I see this as kind
of like with every step that we take with,
in terms of scientific knowledge and,
and, um, and technology, we take away a
part of what we once took for granted.
And, and what needs to
come in place of that is an
understanding of this part, right?
And we are somewhat trailing behind
on our understanding of the impact
that we're having on the world.
The real kind of like visceral, not
rational, but like visceral emotional
understanding of our responsibility.
And I think that this is really the
next step that we grow up as humanity
and we take this responsibility.
Arjé Cahn: Uh, you said AI
engineers or engineers in
general have an outsized impact.
Buster Franken: Yeah.
Yeah.
Arjé Cahn: So, I mean, I, I guess
I can buy into that, but does that
also give people, um, a moral.
Uh, obligation.
Buster Franken: Oh yeah.
With great power comes
great responsibility.
Arjé Cahn: Yeah.
Buster Franken: Yeah.
Uncle Ben, I think said
that from Spider Man.
Yeah.
So I, I, I do believe that, you know,
I, I've always felt like this for
myself that, that I, I mean, I don't, I
don't think it's arrogant to say like,
I, I excelled right in, in school.
I, I found it easy to do these things.
I.
And I always felt like I, if there's
anything, if there's any kind of
direction that is given to me in life,
then it has to be that I use this to
serve the good of life in this universe.
How can we help you with that?
Yeah.
So I want to get to those, to the 1
million AI engineers by 2030, by 2030.
And in the end we need everybody
to, to make doing good the
core of what they're doing.
But, um,
Arjé Cahn: but maybe you start
if we small, maybe, I mean, if
I were an engineer out there
in the field, uh, what do I do?
Go to fruit punch.
ai.
Buster Franken: Yeah, you
can go to fruit punch.
ai, click on platform and you, you're
kept up to date on the, on the,
on future challenges that you can
join as an expert, but really the
most impactful thing, um, is that.
Right now to, to create this, to create
this, uh, you know, this flywheel effect,
we, we have, we've come to a business
model that actually works with, which
is working with these enterprises.
So, so the, the, the key position
that I'm going for now is, is
technical management, right?
I want people that are the IT
managers or the CTO or the head of AI.
That feel like there is, there is
more that I can do here, you know, and
that is, you know, with their teams
and then they're with their teams.
If they want to, if you want to train
your engineers, then why not train them
through Fruitbunch AI, where they're also
making a positive impact at the same time.
Arjé Cahn: What is the one challenge,
your dream challenge that you
would hope to run into one day?
The one that, I don't know, find
a cure for cancer or like what's.
Buster Franken: Yeah.
So we're literally, we've literally
started working on this already.
There's a lot of cures for cancer, right?
There's a lot of specific.
Yeah.
So, so.
Okay.
But I was going to keep it short.
Arjé Cahn: Well, I don't know.
I mean, do you have a challenge right
now that people can sign up for?
Buster Franken: They can't sign up yet.
We're still, we're still in the, but, but
one of these weeks where, where they will
actually be working towards curing cancer.
Yes.
Arjé Cahn: I'm very slow
publishing these podcasts.
So maybe.
Yeah.
So then it's like, but
this is a great example.
So you have.
Challenges that work on
these kinds of things.
Buster Franken: AI for genetics research,
using transformers to, to find, using
transformers and a new compression
algorithm to find, uh, relations between
certain medicine and certain genes
so that we can identify, is this life
saving drug going to work on you or not?
Because a lot of life saving
drugs, they actually only work on
5 percent of the entire population.
And that.
That in, in, in a lot of cases has
to do with a, with a certain set of
genes that people have, but it's,
it's, it's very difficult to find that
as a normal, as a researcher, but a
machine learning model can do this.
So,
Arjé Cahn: so they predict whether
a certain kind of medicine is going
to help the individual yes or no.
Buster Franken: Yeah, yeah, exactly.
So that's literally using AI
to, to, to try and cure cancer.
Yeah.
Arjé Cahn: And you're using
a transformer to do that.
Buster Franken: Yeah, yeah.
Transformers.
Yeah.
Arjé Cahn: So if you could
translate transformer into
normal people speak, it would.
Buster Franken: Yeah.
So it's like these, uh, large language
models, um, like, uh, like chat GPT,
but then instead of input and training
it on a lot of text data, you train it
on a lot of, well, it's actually also
text data because it's ATG, right?
Like it's DNA.
Um, and then that also has a certain,
certain structure and certain
repetitions, just like, you know, words
and grammar and these kinds of things.
So you can actually predict it.
Arjé Cahn: Yeah.
Well, um, thank you.
Let's change the course of
humanity with a million engineers.
Yeah, absolutely.
Good luck, Buster.
Thanks.
Thanks for joining us
at the fire site today.
If Buster's vision of technology as
a force for good resonates with you.
Then, please help us spread the word.
You'll find impact innovators at
the fireside on apple podcast.
Spotify and other major platforms.
Consider giving us a rating.
It helps others discover these
conversations and sharing your
favorite insights on LinkedIn can spark
important discussions, Aero network.
Join us next time as we continue
to explore how technology can help
solve humanity's greatest challenges.
Creators and Guests
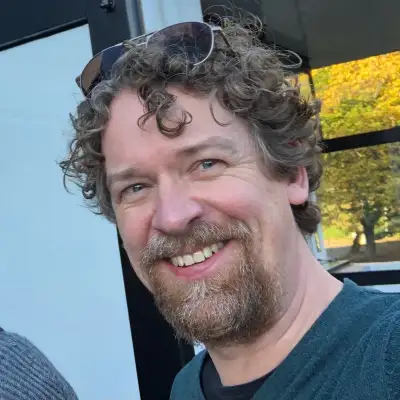
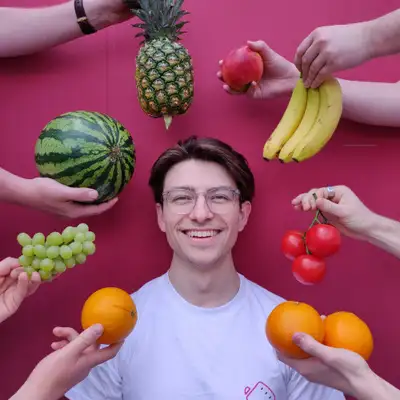
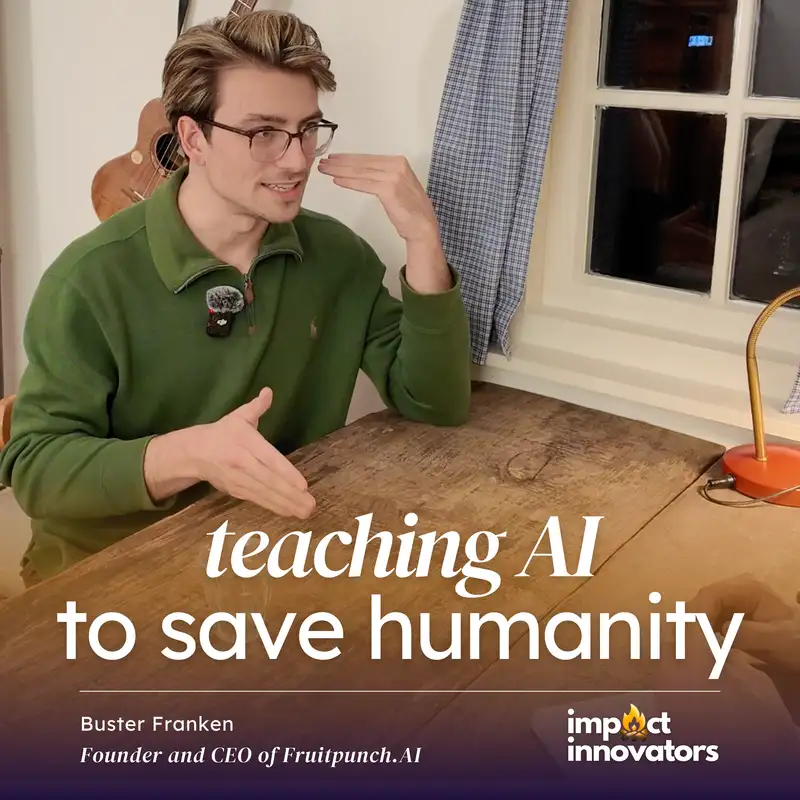